New AI Model Reveals Structures of Crystalline Materials
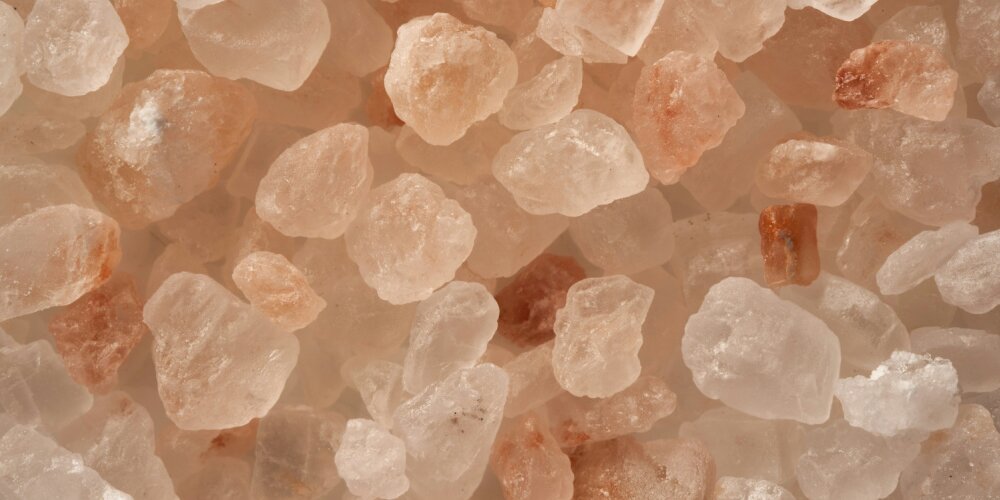
An AI model created at MIT that can predict the crystal structures from X-ray diffraction data could accelerate materials research for applications in batteries, magnets, and other applications. This breakthrough in crystallography, a technique scientists have been using for more than 100 years to determine the structure of crystalline materials, could mark the key to solving structures that have remained unsolved for years.
“Structure is the first thing that you need to know for any material. It's important for superconductivity, it's important for magnets, it's important for knowing what photovoltaic you created. It's important for any application that you can think of which is materials-centric,” said Professor of Chemistry at MIT, Danna Freedman.
Crystalline materials are made of lattices that consist of identical, repeating units. When X-rays are beamed into these lattices, they diffract off atoms with different angles and intensities, revealing positional information about the atoms and the bonds between them.
The new study, which appears in the Journal of the American Chemical Society, was co-authored by MIT graduate student Eric Riesel and Yale University undergraduate Tsach Mackey.
The AI model, called Crystalyze, uses machine learning trained on information gathered on over 150,000 materials from the Materials Project database. The model breaks down the structure prediction process into subtasks.
It begins by determining the size and shape of the lattice, and which atoms go into it. Then it predicts the arrangement of atoms with the lattice. For each diffraction pattern, the AI model generates several possible structures that can be tested by a model that determines diffraction patterns.
“Our model is generative AI, meaning that it generates something that it hasn't seen before, and that allows us to generate several different guesses,” said Riesel. “We can make a hundred guesses, and then we can predict what the powder pattern should look like for our guesses. And then if the input looks exactly like the output, then we know we got it right”.
The model was tested on several thousand simulated diffraction patterns from the Materials Project, as well as more than 100 experimental diffraction patterns from the RRUFF database. The model was accurate about 67% of the time.
Next, the team tested the model on unsolved diffraction patterns from the Powder Diffraction File, which contains more than 400,000 solved and unsolved materials. They discovered structures for over 100 of these unsolved patterns.
“We found a lot of new materials from existing data, and most importantly, solved three unknown structures from our lab that comprise the first new binary phases of those combinations of elements,” added Freedman.
Determining structures of powdered crystalline materials could help work in any materials-related field, according to the MIT team.
Utilise differential scanning calorimetry at The Lab
Here at The Lab, our DSC services can analyse a range of materials and use variations in temperature against time to monitor changes in physical properties of the sample.
To find out more about The Lab at Brookes Bell’s technologies, contact The Lab today.
Discover our differential scanning calorimetry services at The Lab
For more information, industry insights, and the latest news, explore The Lab’s News and Knowledge Hub…
Could Eggshells Be the Solution for Greener Rare Earth Element Recovery? | Scientists Test UV Glass to Disrupt Biofilm Formation in Water | Researchers Find New Way to Weld Metal Foam Without Compromising the Material
- Date
- 03/12/2024